Real-world study: from real-world data to real-world evidence
In recent years, real-world data (RWD) and real-world evidence (RWE) have rapidly become a focus area of medical research and attracted extensive attention from academia, industry and government.
Given the high exposure of these topics, the concept of RWD and RWE have been fully discussed and well defined in many publications (1-3). Literally, it would not be difficult to understand that RWD is the raw materials based on which RWE was generated. However, in the world of scientific research, process matters. A poor research process could easily ruin the valuable study data and lead to inappropriate conclusions and misleading evidence. Therefore, in order to make the best use of RWD to generate the most convincing RWE, special attentions must to be paid to the study process.
In order to have a more objective understanding about this process, this paper shares some facts of as well as thoughts about the process by asking four simple questions. Several terms have been used interchangeably to describe this process, such as real-world evidence study, real-world data study, real-world research and real-world study (4-7). Instead of discussing and distinguishing these terms or coining a new one, real-world study (RWS) would be used throughout this article.
What is RWS?
RWS is not a stand-alone concept. Its definition depends on the understanding of other two highly related terms, RWD and RWE.
Many organizations have developed their version of RWD definition (Table 1). Despite a certain degree of disparity remains, there are no major contradictions among them. All these definitions were defined from the aspects of source or environment from which the data were generated or collected. However, data by itself is useless. The value of data lies in the information and knowledge derived from data. It is not data but these evidences that shape our understanding and guide our practice in real world. US Food and Drug Administration defines RWE as the clinical evidence regarding the usage and potential benefits or risks of a medical product derived from analysis of RWD (8). Similar definition was also adopted by China National Medical Products Administration (9).
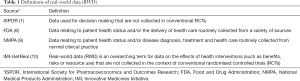
Full table
What is RWS then? In simple words, it is the process from RWD to RWE. To be more specific, it is a research process for a predefined clinical question, and uses study subjects’ health related data collected in a real-world environment or summarized data derived from these RWD to generate evidence regarding the usage and potential benefits-risks of medical products through data analysis (9). It could be categorized into two sub-types, non-interventional (observational) study and interventional study (11).
Why is RWS?
Although it is only recently that the term RWS became popular, its basic concept was not new. Observational study, a key sub-type of RWS, is a major tool of epidemiology research and had been widely used for a long time. In 1849, Dr. John Snow (1813–1858), who is considered nowadays as the founding father of modern epidemiology (12), used observational investigation methodology identified the communication mode of cholera which had been successively outbroken in London since 1831, and successfully subsided the spread of the disease in 1854 (13,14). Moreover, numerous RWSs had already been conducted, reported and published in the area of medical science even before the term RWS was created. Searching common observational study types, such as cohort study, cross-sectional study and case control study in Pubmed.gov will return tens of thousands of results. What make such an old and widely used study type popular again? Two driving forces from internal and external aspects might explain.
Internally, the great diversity and flexibility of RWS make it a perfect tool to address different research questions under various circumstances. Randomized controlled trial (RCT) has been considered the gold-standard for studying causal relationships (15). Based on different study design, RCT could answer only three types of question: (I) Is treatment A better than B? (II) Is treatment A not inferior to B? (III) Is treatment A equivalent to B? However, in real world, the scope of our interest is much wider than causal relationships. Epidemiologists and public health professionals might be interested in the prevalence, incidence and risk factors of different diseases or health conditions, clinical physician might want to know the real world treatment pattern and prognosis of certain diseases, drug administration needs to monitor safety events after a medical product is approved, policy makers needs evidence regarding cost and effectiveness. None of these questions could be properly addressed by conventional RCT. On the contrary, the diversity and flexibility of RWS in terms of its study design and data source enable RWS a powerful research tool while facing these various research questions in real world. It needs to be emphasized that the difference in research question, study design and data sources are not the only distinctions between RWS and RCT, more comprehensive head-to-head RWS/RCT comparisons have been well discussed and can be found in many publications (2,11,16).
Externally, the dramatic environment changes further boost the application of RWS. Firstly, during the past several decades the research and development cost of successfully launching a new drug has significantly increased. It was estimated that the total cost per approved new drug had increased from 179 million dollars during 1970s-early 1980s to 2,558 million dollars during 2000s–mid 2010s (17). Therefore, approaches that could lower the cost of drug research and development would become very attractive. As a type of study design that could utilize existing data, RWS has the potential to significantly reduce the cost of data collection in terms of time and money, which makes RWS a focus of attention. Moreover, as the value of RWE was recognized by regulators, a supportive policy environment was gradually established. During the past 2–3 years, in order to better facilitate and guide the proper use of RWD/RWE in regulatory decision making, several regulatory documents were released (8,9,18,19). April 4, 2019, based on data from electronic health records and post-marketing safety reports, US FDA approved IBRANCE® (palbociclib) for the treatment of men with HR+, HER2- metastatic breast cancer (20). Besides the strong motivation from pharmaceutical industry and the supportive environment created by the regulators, the rapid technology developments in data collection, data storage, data processing and data analysis, further promote the utilization and popularity of RWS. The widely adoption of electronic database in medical services quickly accumulates large volumes of health data in the real world. With the help of optical character recognition and natural language processing technology, complex raw data could be rapidly translated into machine-readable and analyzable data, which again greatly expands the data source for RWS. Furthermore, advances in big data analytic technology provide powerful tools to extract values and insights from huge quantities of data.
How to conduct a RWS?
RWS is essentially a type of scientific research. Therefore, in order to generate solid evidence, it has to strictly follow the universal rules of clinical research. Good Clinical Practice is an international ethical and scientific quality standard for designing, conducting, recording and reporting clinical trials that involve the participation of human subjects (21). Similar standard also exists in the area of RWS. In 1996, International Society for Pharmacoepidemiology developed the Guidelines for Good Epidemiologic Practice, which was revised and superseded by the Guidelines for good pharmacoepidemiology practices in 2004 (22). The fourth version was released in 2015 and is considered as the standard pertaining to the planning, conduct, and evaluation of pharmacoepidemiologic research (23). However, it should be noted that the guidelines only propose essential practices and procedures that should be considered to help ensure the research quality and integrity, but do not prescribe specific research methods nor will adherence to guidelines guarantee valid research (23). The validity of RWS depends on the scientific value of research question, proper study design, valid study data, unbiased data analysis and appropriate result interpretation.
Like any other clinical studies, RWS starts with a research question, which guides and drives the whole design and conduct of the study. Therefore, a clearly defined research question must be determined before any study activities. Research questions of a RWS were usually about the prevalence, incidence, cause/association, treatment effects and prognosis of a health condition. Based on different research questions, different study designs could be adopted. Table 2 gives some RWS designs and the objectives that commonly associated with them.

Full table
After research questions were clearly defined and potential study design was identified, the next step is to evaluate the availability of study data. As RWS could use existing data, it would be always recommended to search for such existing resources first. Examples of these data include electronic medical/health records, claims data, registry data, etc. However, due to the issues of applicability, heterogeneity, accessibility and completeness of these secondary data resources, under many circumstances, researchers have to collect or recollect study data purposefully, either prospectively or retrospectively. It is worthy of pointing out that the process of data collection is also a key component of study design, what data elements from what sources by what personnel using what tools at what time should all be clearly planned at study design stage. Moreover, necessary data management procedure and quality control measures should also be taken to ensure the quality of study data, so that we could smoothly move to the next stage—data analysis.
Presenting valid outcomes for result interpretation is the common goal of data analysis for all study types. Therefore, a pre-specified analysis plan has long ago been accepted as a key requirement for good practice for not only RCT but also RWS. In order to preserve the statistical soundness of overall study design and avoid the risk of increased false positive (type I error), unplanned ad-hoc analysis is usually not recommended in RCT. Although exploratory analysis is more common in RWS, it doesn’t mean researchers could overlook the importance of analysis plan. On the contrary, due to the inherent bias and confounding in RWD, a pre-specified analysis plan describing the methods for analyzing and presenting results, the procedures to control bias and confounding, and the approaches to evaluate their influence on results is essential to ensure the validity of RWS and should not be ignored. Moreover, for a study using existing secondary data, since data analysis would be the main study activity, a pre-specified and well-developed analysis plan is actually the core component of study protocol. Before moving to the next step, it must be emphasized that despite its important role of bias and confounding adjustment, statistical analysis is not and should not be considered as the only way to control bias in an RWS. Comprehensive approaches during study design, data collection, statistical analysis and result interpretation should be taken in order to minimize the influence of bias.
Result interpretation is the progress through which we translate study tables and figures into our understanding and knowledge about the research question. Since it is the step that directly generates evidence, special cautions must be taken. A common manner of result misinterpretation is paying too much attention to the results themselves, in another word, interpreting the results solely based on the significance tests and the corresponding P values. The definition, use and misuse of P value have been well discussed in many publications (24-27), and will not be re-emphasized here again. It should be noticed that, in RWS, no matter what results were obtained, it could be caused and influenced by many factors, including selection bias, information bias, unmeasured confounding, missing value, unmet statistical assumptions, all of which could not be accounted by P value. Therefore, drawing a scientific conclusion or making a policy decision based only on whether a P value passes a specific threshold could be very misleading. A proper interpretation should be cautious and should take the quality of overall study design and conduct into consideration. Knowing the limitations of a study is as important as knowing its values.
What is the limitation of RWS?
Like a coin has two sides, RWS has both strengths and weaknesses which are related closely. From data aspect, the flexibility of data source greatly expands the scopes of RWS. However, the associated increased data complexity might introduce various biases and confounding effects which could influence the study result. For example, in an observational study to evaluate two treatments, there would always have some unbalanced characteristics that were associated with both treatment and outcome. Although certain statistical adjustment methods were available, only measured confounders could be controlled and it was impossible to eliminate them all. Data accuracy and completeness were another two issues for many RWS using existing secondary data source. From study design aspect, retrospective design, such as case-control study and retrospective cohort study, might not be good to answer questions about causal relationships. Results from such study designs only suggest association instead of causation. From analysis aspect, more complicated statistical methods would be required in order to control potential biases inherent in RWD. However, many assumptions which were needed for the validity of these statistical methods were either wrong or hard to prove in reality, which might mislead the understanding of study results. From study conduct aspect, though resources saving is considered as an advantage of RWS, it might not be the case for a perspective design with long duration of follow-up. A large, long-term, well designed and implemented perspective cohort study would be very costly in term of both time and budget. A clear understanding about the limitations of RWS would be extremely helpful for the design, conduct and interpretation of an RWS.
Conclusions
Data by itself is useless. It is the evidence that generated from data through study that reflects the values of data. Driving forces from internal and external aspects greatly boost the popularity and usage of RWS in medical research. In order to generate solid evidence, basic rules and procedures of clinical study must be followed during the design and conduct of RWS. Given the limitation of RWS, special cautions should be taken while interpreting the results from an RWS.
Acknowledgments
Funding: None.
Footnote
Conflicts of Interest: The author has completed the ICMJE uniform disclosure form (available at http://dx.doi.org/10.21037/tbcr-20-19). YW is a full-time employee of Medbanks Group, which is a commercial company specializing in the healthcare and clinical research services.
Ethical Statement: The author is accountable for all aspects of the work in ensuring that questions related to the accuracy or integrity of any part of the work are appropriately investigated and resolved.
Open Access Statement: This is an Open Access article distributed in accordance with the Creative Commons Attribution-NonCommercial-NoDerivs 4.0 International License (CC BY-NC-ND 4.0), which permits the non-commercial replication and distribution of the article with the strict proviso that no changes or edits are made and the original work is properly cited (including links to both the formal publication through the relevant DOI and the license). See: https://creativecommons.org/licenses/by-nc-nd/4.0/.
References
- Garrison LP, Neumann PJ, Erickson P, et al. Using real world data for coverage and payment decisions: the ISPOR Real World Data Task Force Report. Value Health 2007;10:326-35. [Crossref] [PubMed]
- Sherman RE, Anderson SA, Dal Pan GJ, et al. Real-World Evidence - What Is It and What Can It Tell Us? N Engl J Med 2016;375:2293-7. [Crossref] [PubMed]
- Makady A, de Boer A, Hillege H, et al. What Is Real-World Data? A Review of Definitions Based on Literature and Stakeholder Interviews. Value Health 2017;20:858-65. [Crossref] [PubMed]
- Cziraky Mark, Pollock Michael. Real-World Evidence Studies. Applied Clinical Trials. 2015;12. [Accessed June 9, 2020].
- Berger ML, Sox H, Willke RJ, et al. Good practices for real-world data studies of treatment and/or comparative effectiveness: Recommendations from the joint ISPOR-ISPE Special Task Force on real-world evidence in health care decision making. Pharmacoepidemiol Drug Saf 2017;26:1033-9. [Crossref] [PubMed]
- Roche N, Reddel H, Martin R, et al. Quality standards for real-world research. Focus on observational database studies of comparative effectiveness. Ann Am Thorac Soc 2014;11 Suppl 2:S99-104. [Crossref] [PubMed]
- Lipworth B, Kuo CR. Real-World Studies in Infrequently Exacerbating Patients With COPD. Chest 2019;156:415-6. [Crossref] [PubMed]
- U.S. Food & Drug Administration. Use of Real-World Evidence to Support Regulatory Decision-Making for Medical Devices. Available online: https://www.fda.gov/media/99447/download. [Accessed April 3, 2020].
- China National Medical Products Administration. Guiding Principles for Using Real World Evidence to Support Drug Research, Development and Evaluation (Draft). Available online: http://www.nmpa.gov.cn/WS04/CL2182/373175.html. [Accessed April 7, 2020].
- Innovative Medicines Initiative, GetReal Project. Sources of real-world data. Available online: https://rwe-navigator.eu/use-real-world-evidence/sources-of-real-world-data/. [Accessed: April 22, 2020].
- Sun X, Tan J, Tang L, et al. Revisiting real-world study. Chinese Journal of Evidence-Based Medicine 2017;17:2.
- Cerda LJ, Valdivia CG. John Snow, the cholera epidemic and the foundation of modern epidemiology. Rev Chilena Infectol 2007;24:331-4. [Crossref] [PubMed]
- Winterton WR. The Soho cholera epidemic of 1854. Hist Med 1980;8:11-20. [PubMed]
- Paneth N. Assessing the contributions of John Snow to epidemiology: 150 years after removal of the broad street pump handle. Epidemiology 2004;15:514-6. [Crossref] [PubMed]
- Hariton E, Locascio JJ. Randomised controlled trials—the gold standard for effectiveness research. BJOG 2018;125:1716. [Crossref] [PubMed]
- Gyawali B, Parsad S, Feinberg BA, et al. Real-World Evidence and Randomized Studies in the Precision Oncology Era: The Right Balance. JCO Precision Oncology 2017;1:1-5. [Crossref]
- DiMasi JA, Grabowski HG, Hansen RW. Innovation in the pharmaceutical industry: New estimates of R&D costs. J Health Econ 2016;47:20-33. [Crossref] [PubMed]
- U.S. Food & Drug Administration. Use of Electronic Health Record Data in Clinical Investigations Guidance for Industry. Available online: https://www.fda.gov/media/97567/download. [Accessed April 3, 2020].
- U.S. Food & Drug Administration. Submitting Documents Using Real-World Data and Real-World Evidence to FDA for Drugs and Biologics Guidance for Industry. Available online: https://www.fda.gov/media/124795/download. [Accessed April 3, 2020]
- Pfizer: U.S. FDA approves IBRANCE® (palbociclib) for the treatment of man with HR+, HER2- metastatic breast cancer. Available online: https://www.pfizer.com/news/press-release/press-release-detail/u_s_fda_approves_ibrance_palbociclib_for_the_treatment_of_men_with_hr_her2_metastatic_breast_cancer. [Accessed April 6, 2020].
- U.S. Food & Drug Administration. E6(R2) Good Clinical Practice: Integrated Addendum to ICH E6(R1) Guidance for Industry. Available online: https://www.fda.gov/media/93884/download. [Accessed April 9, 2020].
- International Society of Pharmacoepidemiology. Guidelines for good pharmacoepidemiology practices (GPP). Pharmacoepidemiol Drug Saf 2008;17:200-8. [Crossref] [PubMed]
- Public Policy Committee, International Society of Pharmacoepidemiology. Guidelines for good pharmacoepidemiology practice (GPP). Pharmacoepidemiol Drug Saf 2016;25:2-10. [Crossref] [PubMed]
- Schervish MJ. P. Values: What they are and what they are not. Am Stat 1996;50:203-6.
- Chavalarias D, Wallach JD, Li AH, et al. Evolution of Reporting P Values in the Biomedical Literature, 1990-2015. JAMA 2016;315:1141-8. [Crossref] [PubMed]
- Wasserstein RL, Lazar NA. The ASA Statement on p-Values: Context, Process, and Purpose. The American Statistician 2016;70:129-33. [Crossref]
- Greenland S, Senn SJ, Rothman KJ, et al. Statistical tests, P values, confidence intervals, and power: a guide to misinterpretations. Eur J Epidemiol 2016;31:337-50. [Crossref] [PubMed]
Cite this article as: Wen Y. Real-world study: from real-world data to real-world evidence. Transl Breast Cancer Res 2020;1:21.